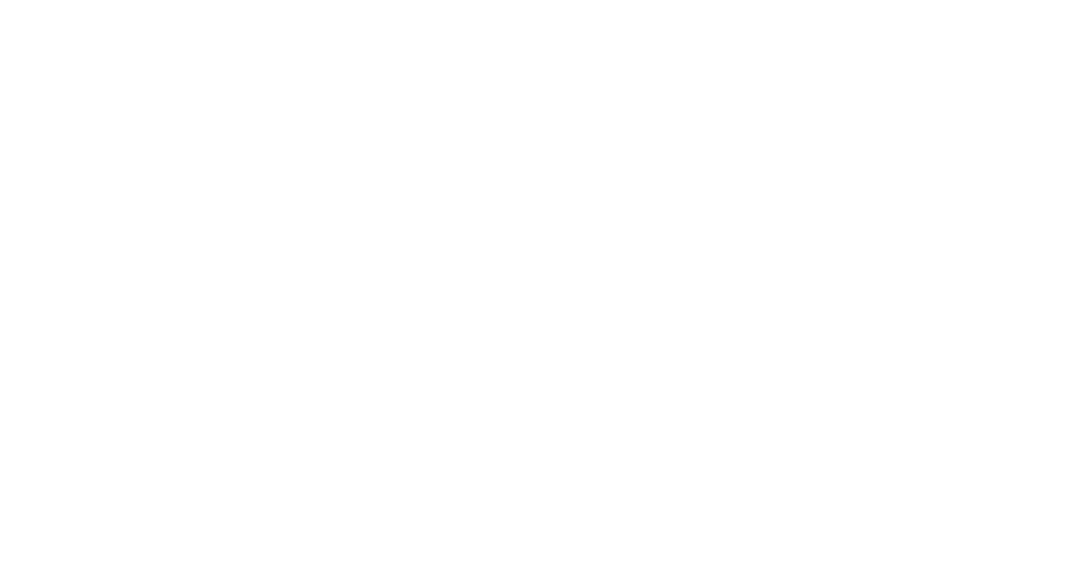
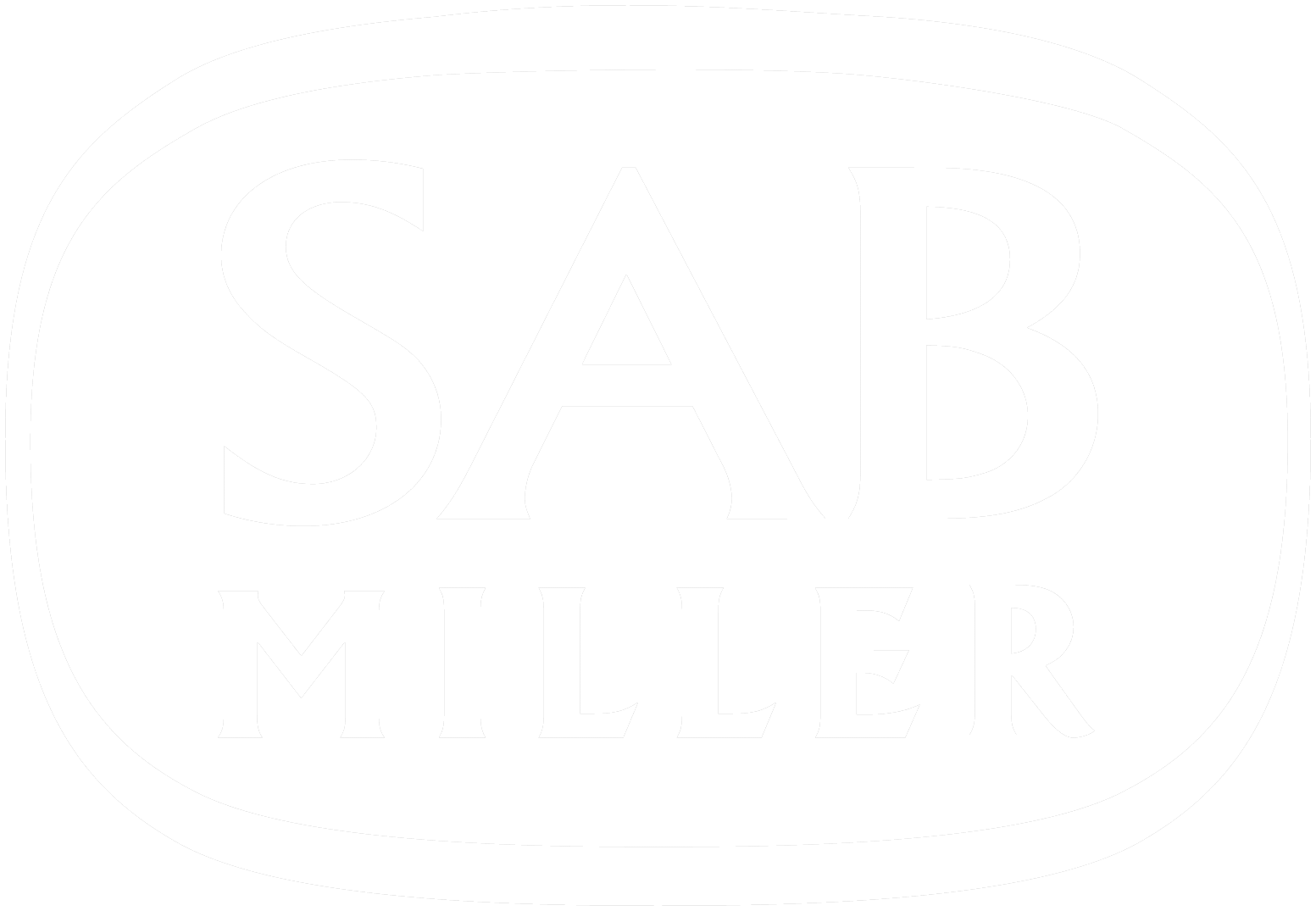
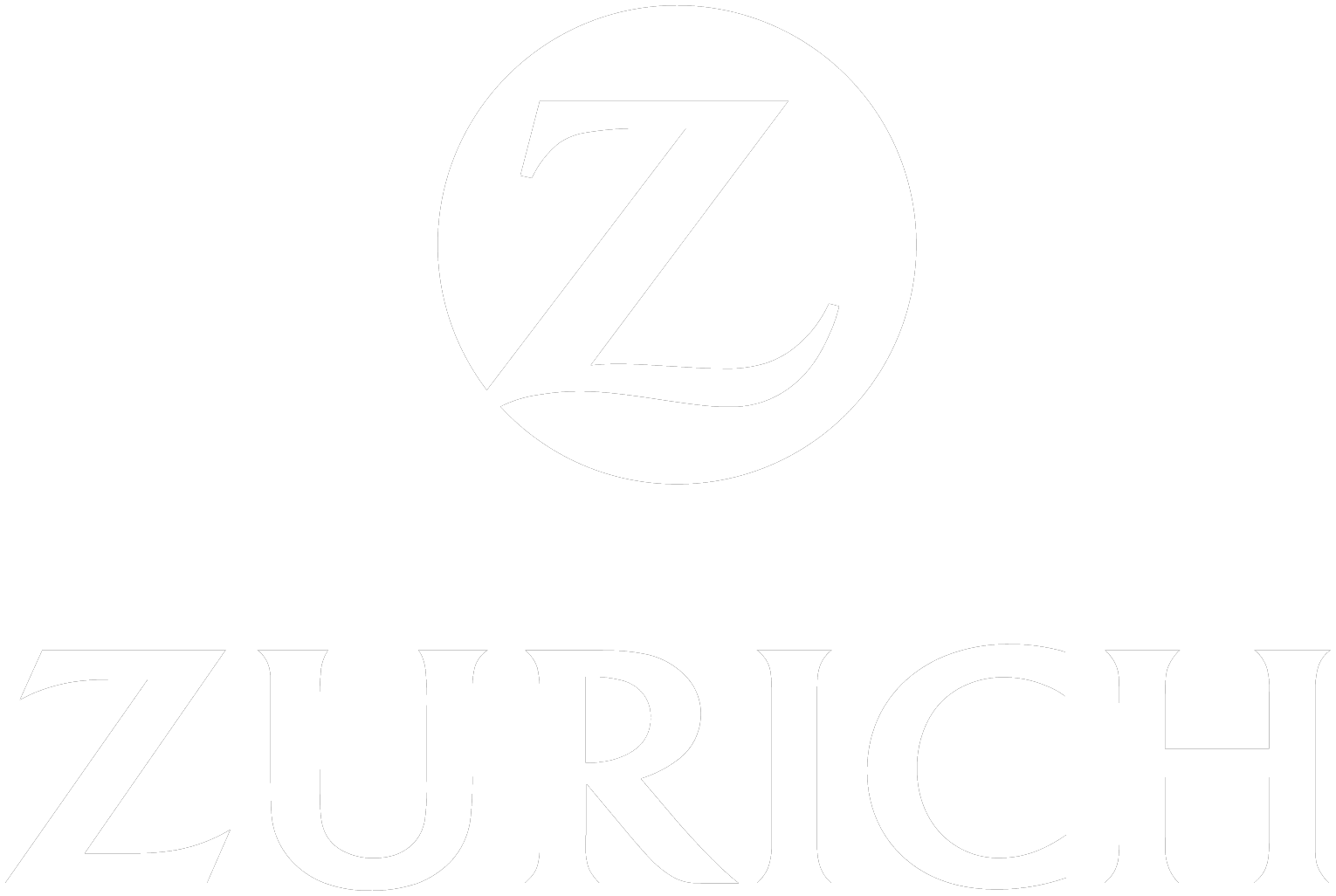
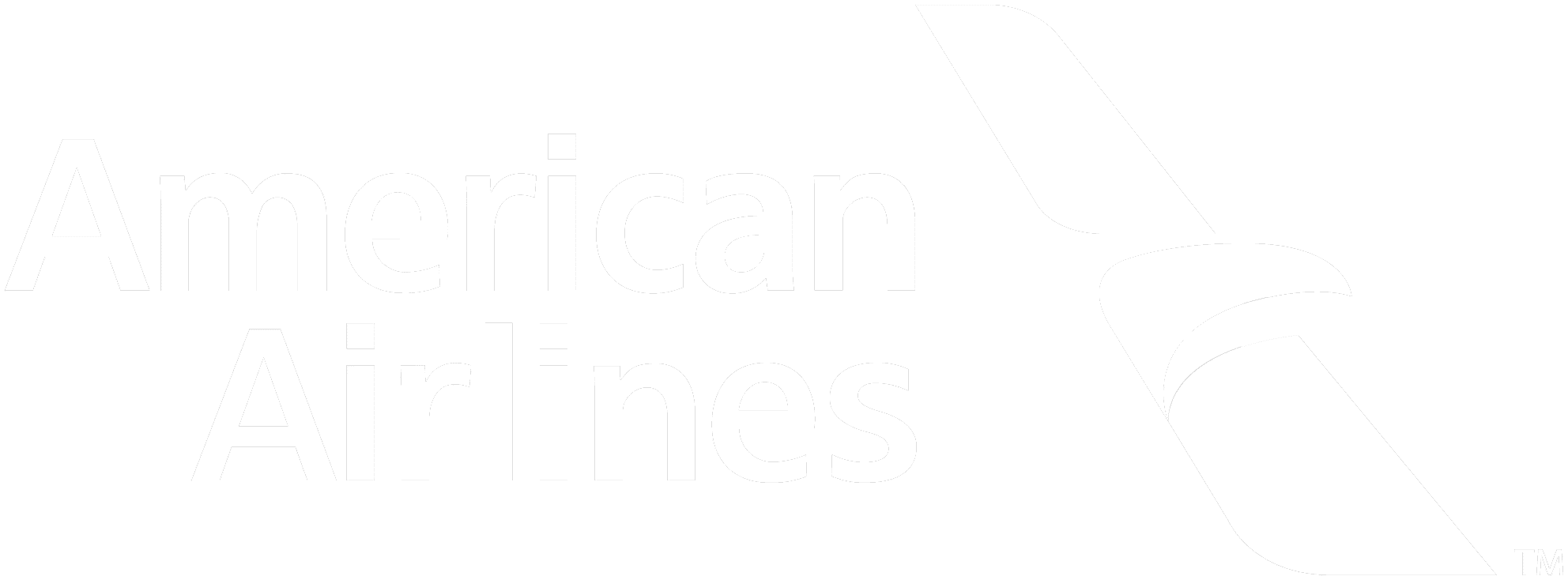
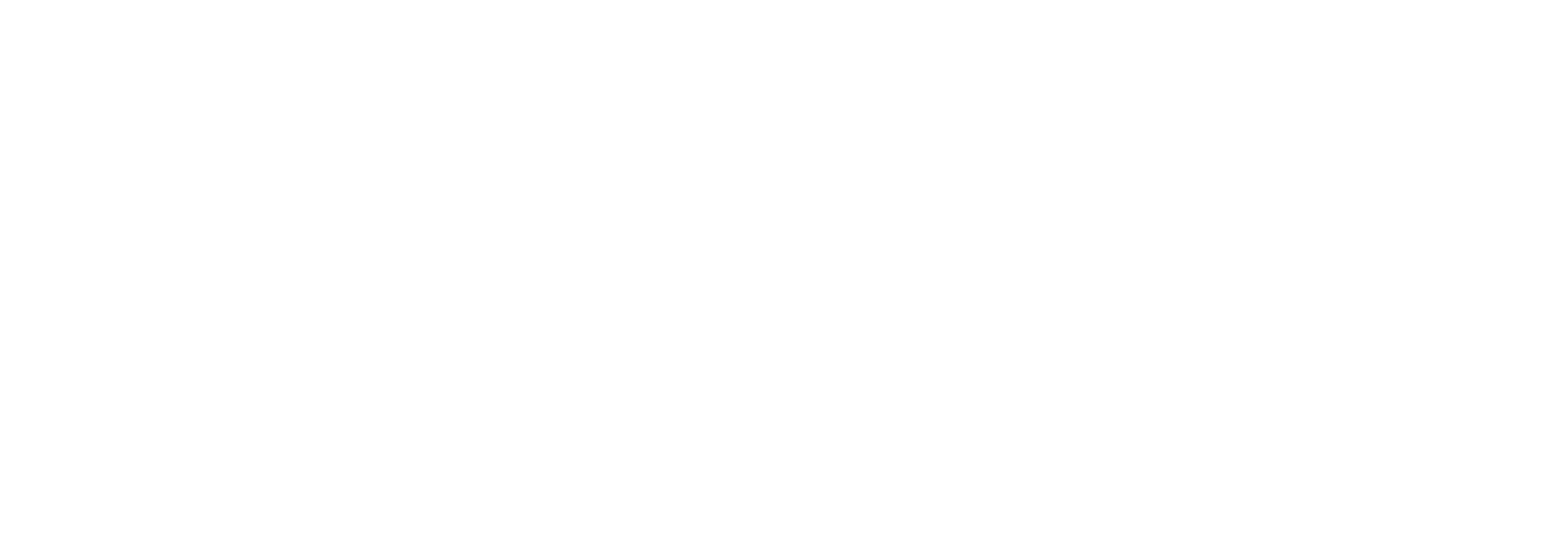
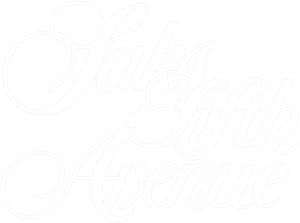
Unlock your enterprise's data: Integrate business strategy with data strategy
Why have several fragmented data management solutions for various initiatives when you could do it all with just one? The Syniti Knowledge Platform (SKP) has data migration, quality, and governance capabilities all in one platform. Unlock trustworthy, optimized, and actionable data — wherever your business requires it.
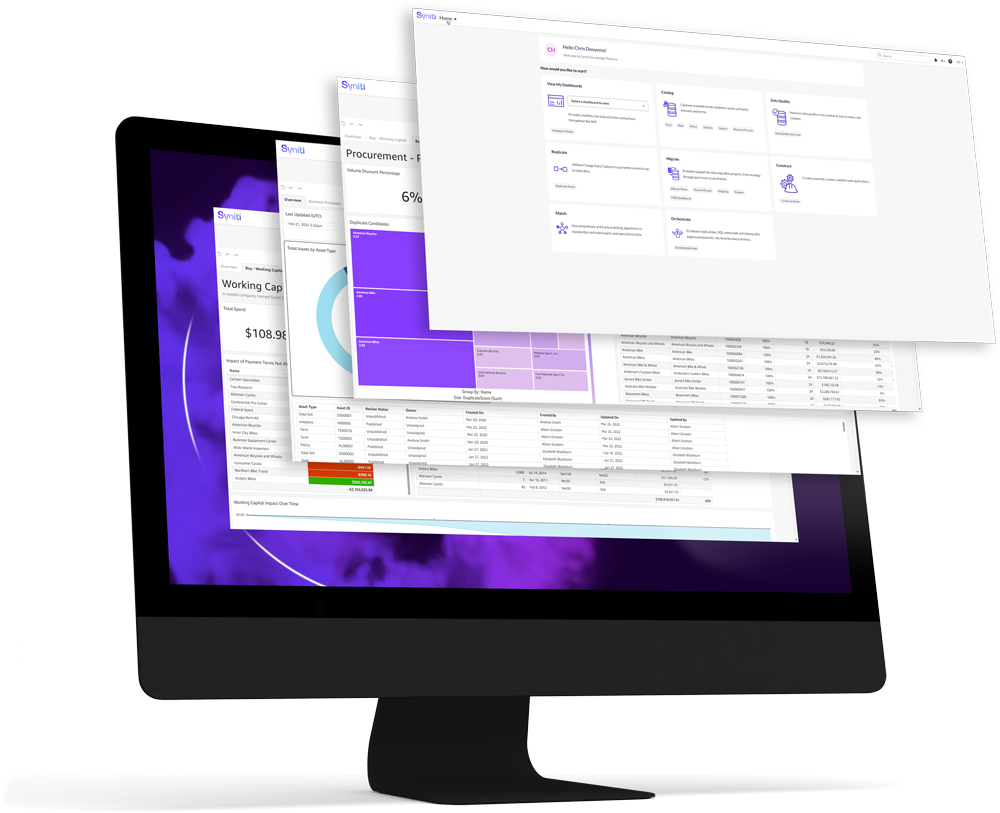
Power the business
Put your enterprise where it needs to be with predictable, on-time, and on-budget data migrations and transformations.
Elevate your data quality and the bottom line
Drive profitability with our suite of data management tools that set a new standard for data excellence.
Cut complexity and accelerate business value
Realize rapid returns with our centralized, cloud-based platform, enhancing data project efficiency by 46%.
Execute with confidence
Tackle transformation challenges with the world's foremost data specialists–100% focused every day on your enterprise’s success.
Lead with data-driven clarity
Power business insights with trusted, accurate, and actionable data.
advanced data migration successes globally
offices filled with data experts across the globe
experts ready to tackle your most complex data challenges
years dedicated to reliable outcomes, deep business expertise, and all things data
The Preferred Solution for Seamless Data Migration to SAP S/4HANA
With a track record of thousands of successful migrations and a 99.7% customer satisfaction rating, Syniti is the trusted partner for getting major SAP data migrations right the first time.
Fortune 500 companies trust Syniti to elevate their strategic capabilities, ensuring a swift and strategic move to SAP S/4HANA. Our approach prioritizes your business continuity, ensuring a seamless shift, minimal downtime, and operational resilience with data optimized for enterprise success from day one.
Ready to RISE to S/4HANA? As a proven leader in Greenfield S/4HANA migrations, Syniti ensures your data and team are perfectly synchronized for a seamless, on-schedule (and boringly uneventful) launch. With our blend of innovative technology, data migration process, and deep expertise, we consistently deliver pristine, compliant, and fully optimized data for SAP S/4HANA.
Transition to SAP S/4HANA with ease, carrying forward your legacy SAP customizations through automated refactoring. Preserve valuable institutional knowledge—our data migration solution retains all rules, roles, policies, and metadata for future use. So subsequent data projects are more efficient, insightful, and impactful.
Put the quality of your data first using Syniti’s Data Assessment Services to rapidly identify costly data challenges ahead of schedule. Within three weeks, gain more traceability and trust in your data so you can move into S/4HANA without disrupting the business.