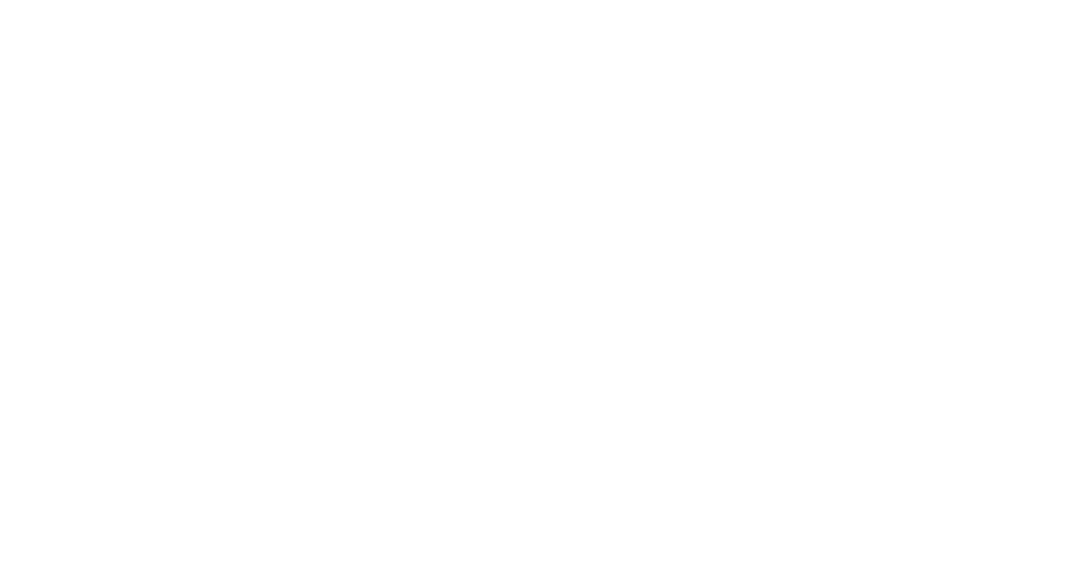
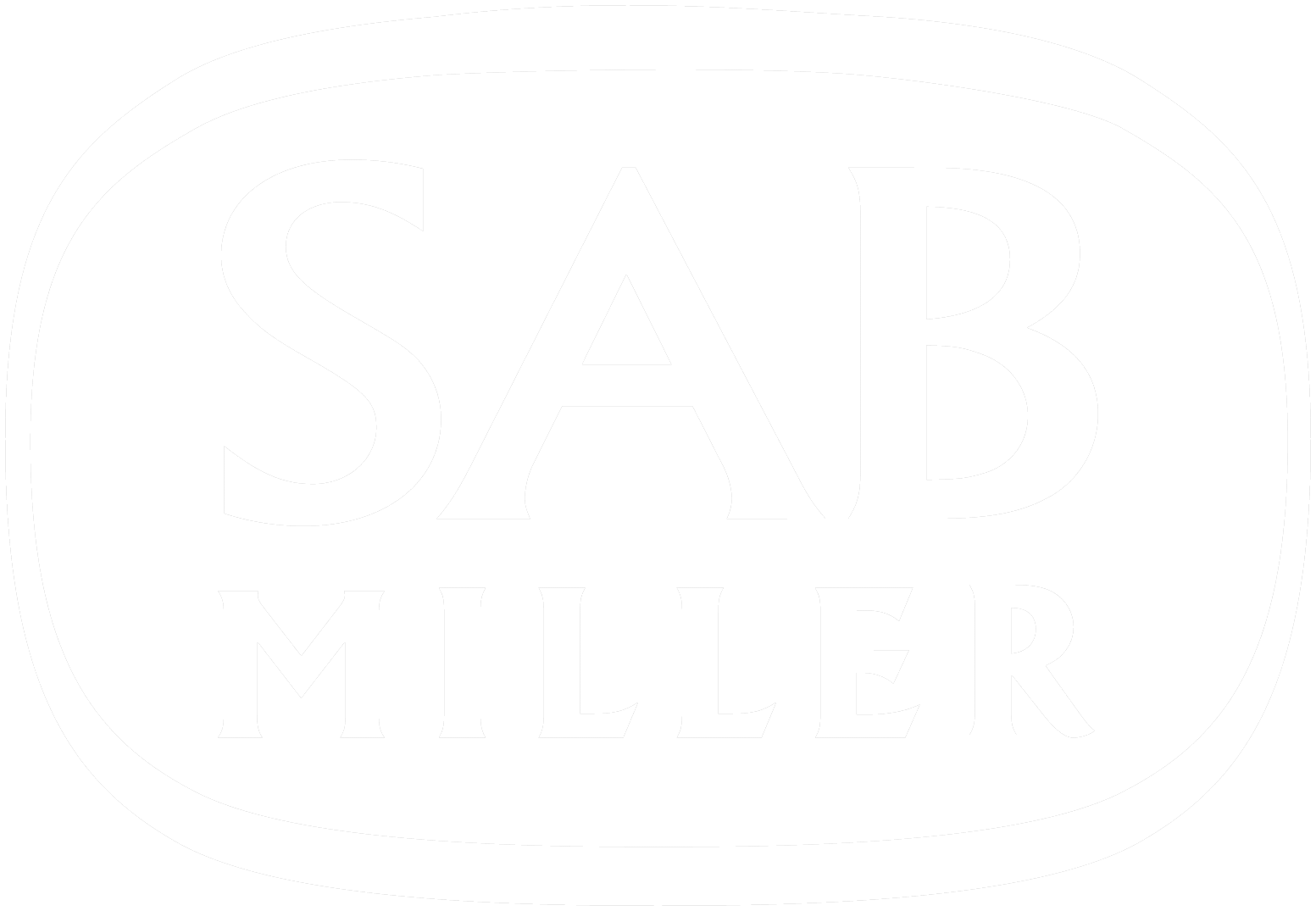
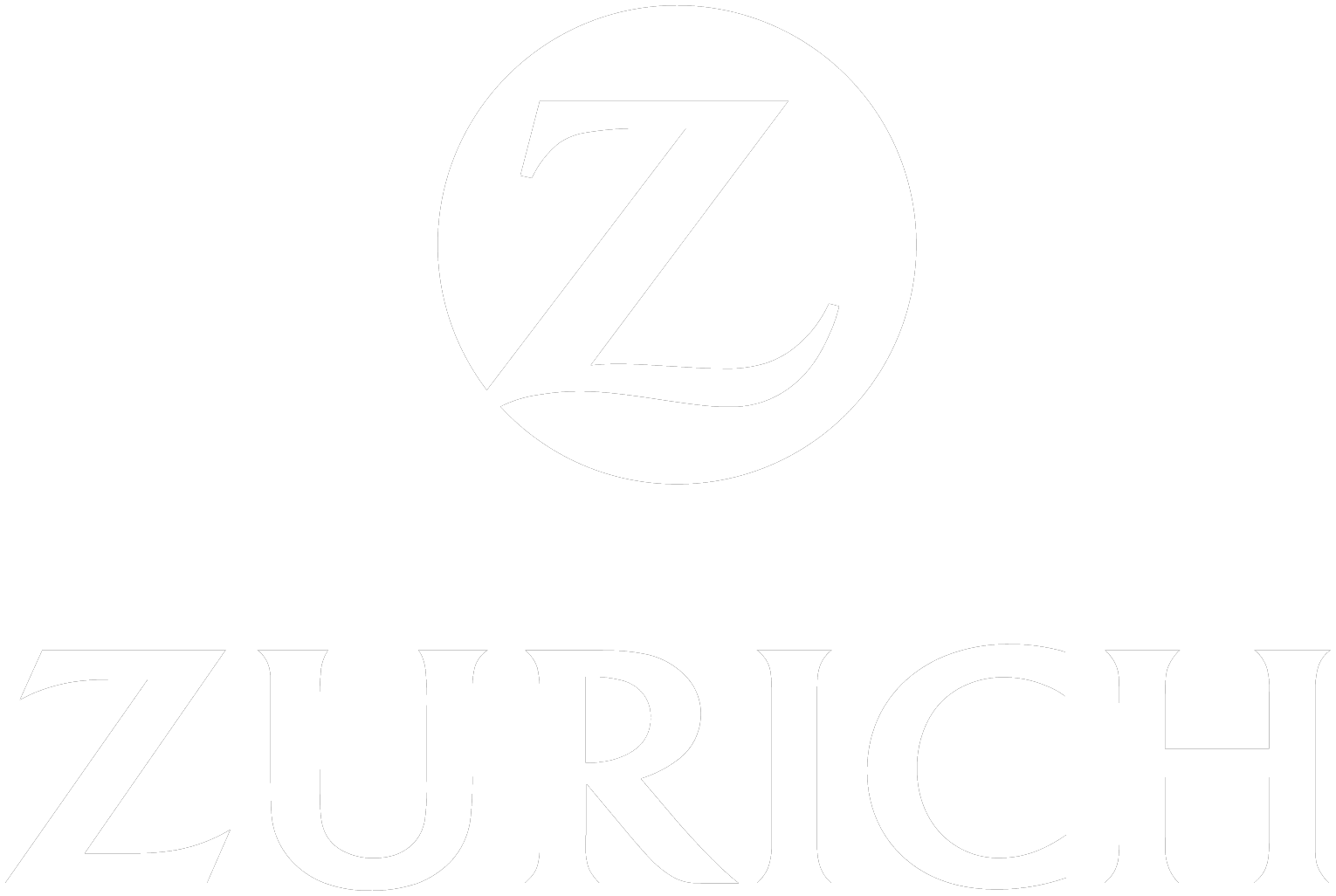
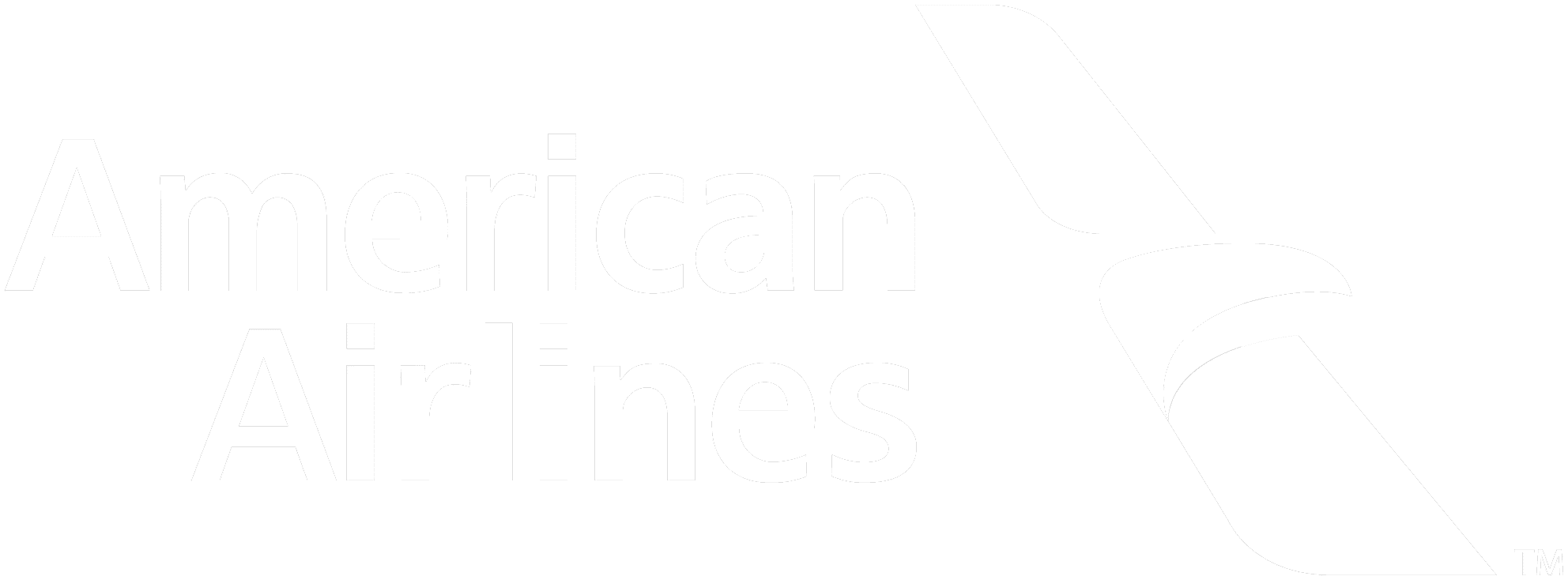
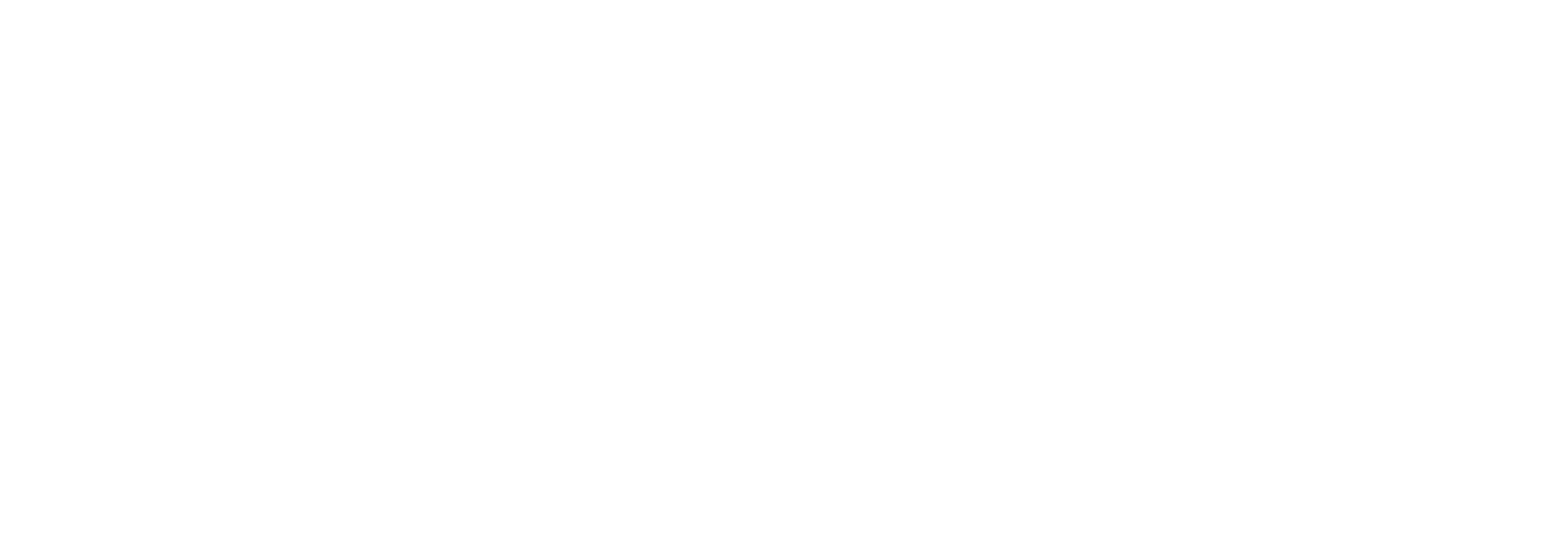
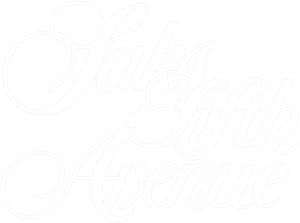
Break down silos with Syniti's enterprise data management platform.
The Syniti Knowledge Platform (SKP) supports your entire team and all your data transformation, data optimization, and data governance needs within a single, unified platform.
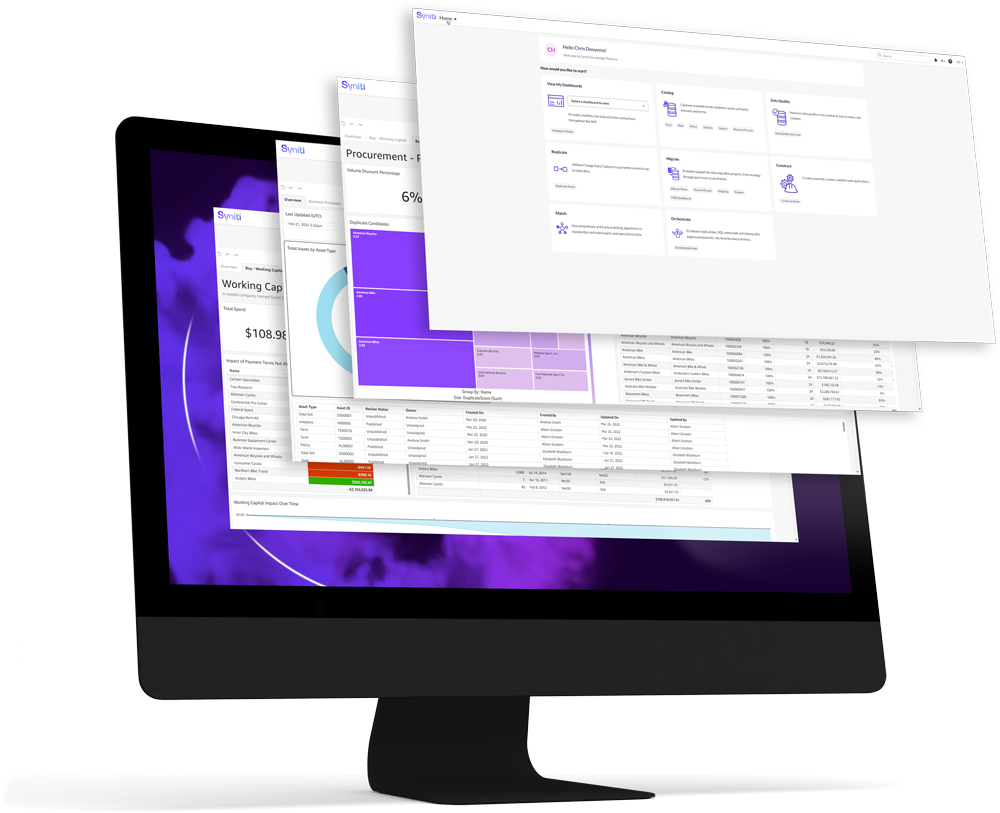
Data ready for digital transformation. Every time.
Transform data flawlessly
Migrate accurate, optimized, actionable data that powers your business wherever your strategy takes you.
Optimize data quality
Benefit from technology and best practices that improve and maintain the quality of your data, resulting in higher profit margins.
Deliver faster time-to-value
Speed up your processes with centrally managed data in one cloud-based platform, and realize a 46% increase in data project efficiency.
Execute with confidence
Solve data challenges with the support of one of the world's largest teams of 100% data-focused experts.
Make faster, more accurate decisions with trustworthy data.
Make faster, more accurate decisions with trustworthy data.
Enterprise-scale data go lives
Global footprint with offices in 30 countries on 6 continents
100% data-focused enterprise data experts
Years solving the world's most complex data challenges
SAP Solution of Choice for Migrating to SAP S/4HANA
Syniti has successfully migrated thousands of enterprises to SAP, and our 99.7% customer satisfaction rating is a testament to getting it done right, the first time.
Syniti delivers on SDT projects to Fortune 500 companies worldwide. Our bundled solution creates a copy of your current systems, removes and optimizes data, updates the application, remediates custom code, and then selectively returns data to you with near zero down time. With Syniti, you start day one in SAP S/4HANA with Intelligent Enterprise-ready data.
Syniti is the established system of choice for Greenfield S/4HANA migrations. Our solution effectively aligns your data and team to orchestrate a stress-free, on-time program. Thanks to the combination of technology, methodology and expertise, we deliver data to SAP S/4HANA that is clean, compliant, and uncompromised–every time.
With automated refactoring of custom code, you’ll be able to bring any legacy SAP modifications with you to SAP S/4HANA. And don’t throw away your valuable knowledge. Our solution captures all rules, roles, policies, and metadata, and saves it for re-use, ensuring future data projects are faster, easier, and more successful.
Learn why Syniti partners with smartShift and NatuvionHow ready are you to move to SAP S/4HANA? Syniti’s assessment suite helps you rapidly gain visibility into your SAP landscape, the current state of your data, and any custom code considerations. In under three weeks, you’ll have confidence in your approach, a budget and risk estimate and a timeline for moving forward.